4 ‘Must Ask’ Questions for a Modern BI and Analytics Strategy
As you are probably aware, the world of data, business intelligence (BI) and analytics has been changing rapidly over the past several years and that trend is expected to continue. It is therefore necessary to look differently and ask some new and different questions when defining a BI and analytics strategy and architecture. In this article, I will outline 4 questions that must be asked when defining a modern BI and analytics strategy. I will not address some ‘old school’ but vitally important topics like, defining your audience, aligning BI objectives to corporate and business unit goals, and understanding the key metrics that drive your business. Those are still necessary and it is assumed that they will be completed. Instead, I will focus on the non-traditional questions that need to be asked. As recently as 2 years ago, these questions might have been optional. But those days are gone. Although I have some strong opinions on the answers, I will only be providing things to consider – not answers.
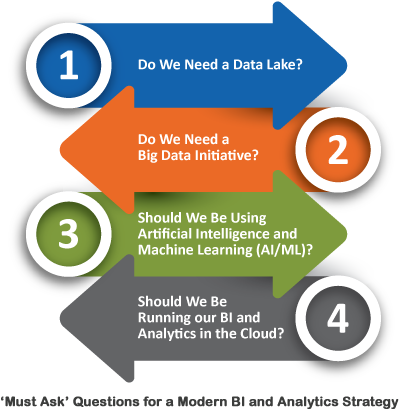
Question #1: Do We Need a Data Lake?
A data lake is a repository that can store any type of data in its raw, native format – structured (e.g. ERP data), semi-structured (e.g. machine sensor data, Twitter activity logs) or unstructured (e.g. photos and videos). So the question that needs to be asked is whether your organization or your business unit or your functional area could benefit from analyzing data beyond traditional structured data in corporate databases like ERP, HCM and SCM. Think about HR using social media comments about the company to help define talent acquisition strategies or Manufacturing using machine sensor data to predict failures before they occur or Sales and Marketing getting a 360-degree view of the customer by analyzing data from mobile devices, sensors and social media. There are many more use cases. Data Lakes are no longer considered fringe or experimental. They are now a mainstream component of modern data management architectures. Data Lakes deserve serious consideration when defining BI strategy and architecture.
Question #2: Do We Need a Big Data Initiative?
This question is related to the first but gets to the question of what will be done with all the data that is collected. It is often stated that Big Data is characterized by 4 main attributes: volume, velocity, variety and veracity. This is true, but the most important point about Big Data is the impact it can have on the business. If a Big Data initiative is to be pursued, it must be executed with a very clear set of goals and objectives. There are endless stories of loosely defined Big Data initiatives that resulted in failure. However, there are also numerous success stories related to well defined Big Data initiatives. It is an important decision and one that should be taken with the proper amount of planning, senior management buy-in, and cross-organizational alignment. Before making the decision, it is a best practice to specifically define the use case(s) where Big Data can have an impact, define the data required, confirm its availability, and start small. Insights gleaned from Big Data can drive competitive advantage so it is also a good idea to get a sense of what your competitors are doing in this area. The Big Data technology ecosystem has matured over the past several years and there are many options available – both on-premises and in the Cloud. So technology risk should not be a deterrent. Big Data initiatives can yield big payoffs if done correctly.
Question #3: Should We Be Using Artificial Intelligence and Machine Learning (AI/ML)?
For many companies, AI/ML can still seem like a far-fetched concept. However, AI/ML is quickly becoming mainstream and there are many vendors that provide AI/ML platforms and capabilities – both on-premises and in the Cloud. That said, to do AI/ML on a significant scale there are some important pre-requisites. AI/ML requires a lot of data to train the models and, in some cases, that data must be labeled through painstaking manual efforts. Appropriate technical environments, platforms, and tools must be made available in a manner that does not conflict with production processing. Also, skilled data scientists with a high level of expertise are required to build and train models and interpret the results. Because there is a certain ‘exploratory’ aspect to AI/ML, sometimes results are not realized right away or in the expected timeframe. There is a bit of an AI/ML ‘arms race’ going on right now that is generating FOMO (fear of missing out). Still, with all that said, successfully applying AI/ML techniques can be a game changer, can redefine business models and modes of customer interaction and can allow a company to leapfrog the competition. So, the AI/ML question deserves serious consideration. (Note: some vendors provide prepackaged AI/ML models for use by business analysts – all that is required is to supply the data. So it is possible to start out very small in this space if desired).
Question #4: Should We Be Running our BI and Analytics in the Cloud?
The Cloud continues to mature and the benefits continue to become more tangible and realizable. As a result, for many companies this question has become a matter of when and not if. Still, the migration to the Cloud is in the early stages, particularly for BI and Analytics. The investment in on-premises data warehouses and related BI platforms is significant and deciding whether to move to the Cloud is a very real and present tense question. Perhaps it is best to outline some of the important characteristics of the Cloud as factors to consider:
- Cloud is elastic – you pay for what you use.
- Cloud is instantly scalable – if you need more processing power or storage it is added instantly – no wait time.
- Cloud requires no upgrades, patching or maintenance – the Cloud providers handle these.
- You can fail fast in the Cloud – spin up the infrastructure you need, use it, terminate it when done.
- The major cloud providers all provide services tailored to Data Lakes, Big Data and AI/ML – up front investment for these critical capabilities is much less than on-premises.
- Cloud providers have made great strides forward in security.
Some other factors to consider when thinking about migrating BI to the Cloud include:
- Where are the source systems? Will all the source systems continue to be on-premises or will it be a mix of on-premises and Cloud?
- What is my company’s strategy with regard to the Cloud?
- Are there any regulatory barriers to prevent moving to the Cloud?
Lastly, if the answer was ‘yes’ to any of the first three questions in this blog post (i.e. Data Lake, Big Data, AI/ML), then it should be noted that it is quicker and more cost effective to get started in the Cloud than to build out on-premises infrastructure.
The competitive landscape continues to heat up and the effective use of data and analytics to gain a competitive advantage is becoming a ‘must have’ capability. We encourage you to consider the questions in this article as you pursue your BI and Analytics strategies.